Quantitative Analysis Tools for Phytosanitary Measures: a Perspective
from South America
Ricardo B. Sgrillo
Cocoa Research Center (CEPLAC/CEPEC)
Rod. Ilheus-Itabuna, km 22, 45650-000 Ilheus, BA, Brazil
ricardo.sgrillo@terra.com.br
This paper represents the views of the author and not necessarily those
of his country and/or organization.
Presented as invited paper at:
INTERNATIONAL
CONFERENCE ON SANITARY & PHYTOSANITARY (SPS) RISK ASSESSMENT METHODOLOGY
"Optimization of SPS Regulatory Tool-Box"
August 9 - 11, 2005
Washington DC
Download Paper (.pdf)
Download Presentation (.pdf)
Summary
This
paper presents some concepts and quantitative tools that could be used
for the development of phytosanitary regulations for plants and plant products.
These concepts could increase biosecurity, reduce unjustified trade barriers
and add transparency to the regulation process. Equations are shown that
give meaning to the Acceptable Level of Risk concept through which it is
established an acceptable probability level for pest introduction. Some
aspects regarding application of phytosanitary measures to reduce the probability
of pest introductions to an acceptable level, either by the reduction of
pest prevalence and/or establishment probability or through sampling for
detection of non-compliant shipments, are analyzed.
Keywords:
biosecurity, quarantine, phytosanitary regulation, pests
1.
Introduction
The
World Trade Organization Agreement on the Application of Sanitary and Phytosanitary
Measures (the SPS Agreement) emphasizes the importance of a sound technical
basis for measures affecting trade. In spite of the recognition of this
concept in the SPS Agreement, very little has been done regarding the development
and application of analytical methods establishing quantitative parameters
for phytosanitary measures based on fundamental mathematical concepts and
statistical analyses.
The
increasing development and application of international standards associated
with the SPS Agreement and increasing pressure by trading partners for
greater transparency and recognition of equivalence has led to a shift
in the paradigm of past approaches. This has resulted in greater interest
and need for appropriate quantitative methods to measure, assess, and justify
risk management decisions.
In
spite of efforts done by IPPC and by many countries, in the last years,
the rate of introduction of quarantine pests is increasing as can be seen
in Figure 1 ([1])1 Probably these figures underestimate the
real number of introduced pests, but they clearly show a trend to increase.
What
worries more are that the rate of pest introduction (Fig. 1A) is
growing and that the number of introduced pests seems to be exponentially
increasing (Fig. 1B). The question that naturally arises from the analysis
of the graph is: how can we revert the growing tendency of pest introductions?
Considering
that is not acceptable to increase barriers to trade, the reduction in
the rate of pest introduction could only be achieved increasing the technical
capacity of the countries.
This
can be shown through a conceptual model of the Global Phytosanitary System,
as presented in Figure 2, and explained by the following hypotheses:
-
1.An increase in the intensity of the international trade tends to increase
the rate of introduction of quarantine pests;
-
2.When the number of introduced pest increases, the cost of commodity production
would also increase (after a few years time delay, because new pest management
techniques will have to be applied and also the crop yield would be reduced).
Higher production cost and lost of yield implies in higher prices, what
put pressure to slow down the rate of international trade;
-
3.On the other side, when the introduced pests quantity increases, the
number of phytosanitary requirements will decrease, which would speed up
the international trade rate by removing trade barriers;
-
4.There is a "natural" build rate of the technical capacity of the countries.
However the increase of the international trade intensity tends to speed
up the technical capacity build rate. This is because the countries need
to defend from the growing phytosanitary risk associated with trade increase.
An increase in the pest introduction rate has the same effect in the build
rate of the technical capacity.
-
5.Improved phytosanitary requirements would be developed and applied when
the technical capacity is increased. This would increase the biosecurity
and, as a consequence, decrease the pest introduction rate. Also, better
phytosanitary requirements imply in the removal of those requirements that
are not technically justified. This would help to increase the trade intensity
by removing unjustified barriers.
-
6.The increase in the people's international transport rate shall also
be considered in the system. It will cause an increase in the pest introduction
rate.
Figure 2. Conceptual model of the Global Phytosanitary System (causal
loops diagram)
A simulation model derived from the conceptual
model generates some interesting figures. We simulated two scenarios: use
of 10% of the potential build of the technical capacity of the countries
(dot line) and use of 90% of this potential build (continuous line). The
results are presented in Figure 3.
It
is clearly displayed in Figure 3 the urgent need to improve the technical
capacity of the countries, which implies that not only is necessary to
increase the use of the available quantitative methods for phytosanitary
management but also that there is a need to develop new methodologies.
In
recent years, the evolution of phytosanitary approaches for the management
of pest risk has led to the recognition of a rationale: if you decrease
the number of fertile pest individuals in a commodity to a pre-established
number assessed through risk analysis, then you will decrease the risk
of establishment of this pest to an acceptable level. A considerable
effort and significant progress have been made through the application
of this rationale to understanding options for achieving a desired level
of quarantine security. This has opened the door for expanding the understanding
and use of many important concepts for risk management, including:
Pest
Free Areas (places and sites of production) - a risk management option
based upon a sound pest risk assessment coupled with satisfactory evidence
of effective, on-going surveillance and exclusion measures to maintain
such areas pest free [2].
Systems
Approaches - consecutive phytosanitary measures are used to decrease
the number of fertile pest individuals in a commodity.
Alternative
Treatment Efficacy - where the percentage of the pest population that
has to be killed is variable, depending mainly on the initial infestation
level and on the characteristics of the pest and of the commodity.
Maximum
Pest Limits (especially for fruit flies) has developed as "the maximum
number of immature fruit flies that may be present in consignments imported
during a specific time to a specific location" (see [3] and [2] for a deeper
discussion on these concepts).
These
initiatives demonstrate a trend toward exploring and applying more systematic
approaches to evaluating (and justifying) the phytosanitary measures. Yet
a common understanding of the range of possible quantitative methodologies
and the development and routine use of appropriate methodologies to evaluate
and compare the risk mitigation measures is far from being well developed
in the phytosanitary community.
2.
Acceptable Level of Risk
As
there is no a risk definition in the FAO glossary, we will adopt a commonly
accepted definition [4]2 where risk is the product of the consequences
of an event by the probability of it occurrence:
Risk
= Consequences * Probability of occurrence 1
In
the phytosanitary context risk would be:
Risk
= Potential Economic Impact * Probability of Introduction 2
In
this context “Introduction” means the entry and establishment of a pest.
However, the probability can be expressed as the time lag between expected
occurrences of the event. If the probability of introduction is a per year
probability then we can express the risk as:
3
With
equation 2 or 3 we can apply the Iso-Risk concept proposed by [5] and expressed
in Figure 4.
Figure 4. Iso-Risk lines (graph in log scale).
Each
line is an iso-risk line, where the combination of Potential Impact
and Probability of Introduction is constant. Each one could represent
the Acceptable Levels of Risk (ALR) for different countries. Notice
that a pest with high introduction probability and low potential impact
could put the same risk that a pest with low introduction probability and
high potential impact.
The
main point to note at this stage is that although countries have the sovereign
right to choose their ALR. It is difficult to understand what this
means, unless it is expressed quantitatively (equations 2 or 3). This is
consistent with the principle of transparency.
If
a pest presents a risk greater than the ALR, there are two ways
to decrease it. Either the Potential Impact is decreased or the
Introduction
Probability is decreased (or both).
Although
there are ways to decrease the potential economical impact, as to begin
cropping resistant varieties or to develop and apply contention plans,
for instance, this paper will keep the focus in the reduction of the Introduction
Probability.
If
the country choose an ALR and have an estimate of the Potential
Economic Impact, then for each pest can be calculated the acceptable
Introduction
Probability, as shown in equation 4. This is essential to support the
choice of a risk management strategy, as will be discussed in the next
section.
4
3.
Probability of Introduction
From
the field in one country to establishment in another country there is a
chain of events that reduces the pest prevalence in one consignment of
a plant or plant product, as illustrated in Figure 5.
Figure 5. Population losses in the production/commercialization chain
For
phytosanitary purposes, it is convenient to have a holistic approach to
this system. For example, a post-harvest treatment with a known mortality
rate has no meaning if we do not know the initial prevalence in the treated
consignment, because we don’t know how many individuals will survive. Also,
the design of an inspection system could be optimized only if we know the
probabilities associated with pest entry and establishment. It is not logical
to design a very sensitive, expensive inspection system to detect a very
low prevalence if the pest has a poor chance to become established.
The
establishment of a pest has essentially two components:
the
number of pest individuals in that enters the country (consignment size
* prevalence), and
the
probabilities of establishment of the pest.
The entry of
a pest does not mean introduction (establishment). Many factors, as described
in [6] could interact to allow, or not, the establishment, as in the following
examples:
-
probability of pest surviving existing pest management procedures;
-
probability of transfer to a suitable host;
-
probability of the environment be suitable in the PRA area, etc.
Equation 5
estimates the expected number of establishments:
Expected
Introductions = Entered x Probability of Establishment 5
To calculate
the necessary efficacy of any phytosanitary measure, it is required the
estimate of the number of individuals that could enter the country without
resulting in establishment, considering the chosen protection level. For
that it is necessary to calculate the introduction probability resulting
from the individuals that enter, and also considering the establishment
probability of the pest. There are equations for estimates of this probability,
as a function of the entered individuals, of the pest prevalence and of
the establishment probability [7]:
6
However as
the introduction probability can also be estimated through equation 5 (because
the values of expected establishments are almost the same to the introduction
probability, when the values are low) this equation will be used, to keep
the simplicity of this work.
Now
it is possible to join equation 4 and 5 according to the following expression:
7
We can plot
now the iso-introduction line in function of the probability of establishment
and of the number of entered individuals, as shown in Figure 6.
Figure
6. Iso-introduction line in relation to the number of entered individuals
and the probability of a single individual establishment. In this graph
the iso-introduction line is set to 0.00001 what means that the chosen
ALR is 100.000 smaller than de Economic Impact. The line represents cases
where
Entered
Individual * Probability of Establishment = ALR/Economic Impact (Probability
of Introduction).
In
Figure 6, a pest is represented by the dot. As this pest is above the iso-introduction
line, it has a risk greater than the ALR. To decrease its risk we have
to either (a) decrease the number of entered individual; (b) decrease
the probability of establishment or (c) detect consignments with
larger prevalence than the acceptable (or any a, b and c combination).
Note
that the lines a and b represents the necessary efficacy
of equivalent measures. Note also that a and b have the same
size but that any other line from the pest to the iso-introduction line
is shorter than a and b. This means that when two measures
are applied the combined efficacy can be lower than the efficacy of a single
measure.
Example 1
Data:
Acceptable Level of Risk: US$ 1,000
Potential Economic Impact: US$ 10,000,000
Consignment size: 10,000 units
Expected Infestation Level in the consignment: 0.005 (0.5%)
Pest Establishment Probability: 0.00001
Results:
Acceptable
number of entered individuals (eq. 7): = 0.0001/0.00001 = 10
Expected
number of entered individuals(eq. 5) = 10,000*.005 = 50 individuals
Conclusions:
Consignments of the product could be accepted if:
1.
It is feasible to apply an inspection plan to detect 0.1% of infestation
level
2.
The infestation level in the consignment could be decreased to less than
0.1%
3.
The pest establishment probability could be decreased to less than 0.000002
4.
Phytosanitary Measures (PMs)
The phytosanitary
measures can be grouped according to their strategy:
Group
1. Reduction of the pest population in the consignment (prevalence)
The
strategy of this group is to reduce the population of the pest in the consignment
and consequently, to reduce the possibility of establishment. This can
be achieved by treatments or other procedures.
-
Pre Harvest (treatment in the field, pest free area, place or site of production,
testing, etc)
-
Harvest (removal of infested products, inspection for selection, stage
of ripeness/maturity, etc)
-
Post Harvest (handling, chemical/physical treatment, etc)
-
Shipping and distribution (volume, transport environment, in transit or
on arrival treatment, limits on distribution, etc.)
-
Pest Free Areas (sites and places of production)
Group
2. Reduction of the probabilities of establishment
The strategy of the second group of PMs is to reduce the
probabilities of establishment and so reduce the probability of introduction.
This group includes measures using import management:
-
Frequency of importation
-
Season timing
-
Port of entry
-
Restriction on the end use
Group
3. Detection of infested consignments
The strategy of measures of the third group is to detect
the consignments that present infestation (prevalence) above an established
threshold. After the detection, the destruction, re-export or another PM
of the other groups (treatment, restriction of the end use) can be applied.
The following measures fall into this group:
Most
of these measures are well studied and applied. There are, however, few
points that deserve additional consideration.
4.1
Reduction of the population of the pest in the consignment (prevalence)
Decreasing
the number of entered individuals has been the main strategy used in the
past and present. The main quantitative application widely used
by the phytosanitary community in the past is the probit 9 approach. It
was proposed more than 65 years ago as the basis for measuring the efficacy
of treatments for fruit flies [8]. The general rationale behind the probit
9 approach is very simple and straightforward: if you kill a
very high percentage (99.9968 %) of the pest population then you will decrease
the chance of pest establishment to zero (or very close to zero). This
rationale assumes a worst-case scenario and results in a high-kill treatment
requirement that may not be technically justified based on pest prevalence.
However
it is interesting to note that the countries usually request the application
of specific phytosanitary measures, but they don't requires maximum population
thresholds. According to the preceding paragraph the number of expected
establishments is directly proportional to the population entered. The
efficacy of a chemical treatment, for instance, has no meaning, if the
initial population size (prevalence) is unknown and consequently the number
of survivals is also unknown.
Figure
7. Necessary treatment efficacy (probit) to reduce the survivors to one
individual, as a function of the initial prevalence in the product, for
different consignment sizes. The dashed line represents probit 9 mortality.
Figure
7 shows that the only cases that requires probit 9 to achieve a resultant
population of one individual is the ones that have an initial prevalence
of 0.31 (31% infection rate) or greater and the consignment size is 100,000
units or greater. All the other combinations need inferior probit. The
excess mortality rate that would be attained with probit 9 in the other
cases do not lead to additional safety and is expensive, could reduce the
shelf-life of the product and increase the residuals concentration (Fig.
7).
Also
the probit 9 approach has been criticized, in particular because: "While
probit 9 was clearly designed to reduce the prevalence of pests by a predictable
amount, it does not account for other variables contributing to pest risk.
Natural survival rates, the likelihood of infestation, and the colonization
potential of the pest are a few of the more important risk based considerations
that are ignored by a direct estimation of mortality such as probit 9.
Process parameters such as pre-shipment cultural practice, packing and
shipping procedures, and distribution times or areas, are not considered
when mortality is the sole criterion for determining quarantine security
[2].
Note
that the required probit can be estimated with equation 8 (from equation
7) and is represented by line b in Fig 6.
8
In
reducing the prevalence there are cost issues that need to be considered.
The cost to develop and apply phytosanitary measures usually increases
with the efficacy of the measures, while the use of measures with low efficacy
could imply in lost of market opportunity and increased cost of opportunity
lost. Figure 8 represents the relation between these costs and the composed
cost.
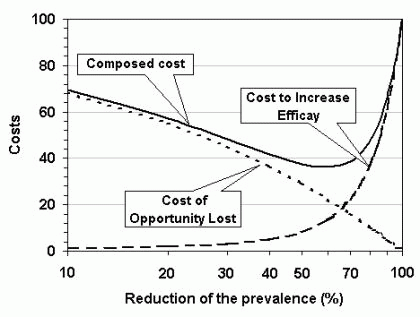
Figure
8. Cost/Benefit analysis of the phytosanitary measures to reduce prevalence.
Figure
8 shows that above certain level it is not economically viable to increase
the efficacy of measures to reduce prevalence. In such cases, it will be
important to evaluate the possibility to use other procedures to reduce
de probability of introduction.
4.2
Reduction of the probability of establishment
Although
it is relatively easy to calculate the required efficacy of phytosanitary
measures to reduce the prevalence is quite more complicated to do estimates
of the probability of establishment of pest populations, entered in a new
environment.
The
establishment probability almost always depends on the size of entered
population. It is interesting to note the observations of [9]: Founder
populations are typically small and consequently are at great risk of extinction.
Generally, the smaller the founder population, the less likely is establishment.
Though many scientists have referred to a “minimum viable population” there
is rarely a distinct threshold. Instead it is more realistic to consider
the probability of establishment as being a continuous function of the
initial population size. This function reflects many characteristics of
the species, such as its intrinsic rate of reproduction, mate location
abilities, and genetic diversity.
This
is in agreement with the statement of Dr. Alexei Sharov (Virginia Polytechnic
Institute and State University - personal communication), who said that:
“… in many cases, the dispersal pattern and the probability of finding
a mate are critical for pest establishment. Insects that mate before dispersal
have a higher probability of establishment. In the destination area, the
initial population numbers are extremely low. So if insects disperse first,
then they will probably never find a mate. This is true even for insects
with very sensitive pheromone communication mechanisms (e.g. gypsy moth)”.
In
1981 it was proposed [10] the concept of Minimum Viable Population (MVP):
An
MVP for any given species in any given habitat is the smallest isolated
population having a 99% chance of remaining extant for 1000 years despite
the foreseeable effects of demographic, environmental, and genetic stochasticity,
and natural catastrophes.
Lately
this concept gave origin to the Population Viability Analysis (PVA) methodology
that consists of estimating the probability of a population of a specified
size that will persist for a specified length of time. The topic of PVA
has become very popular, with two recent books [11, 12] providing extensive
coverage of the topic.
PVA
is usually developed through probabilistic simulation models. Although
models for PVA have been developed for populations of vertebrates, they
could also be used for invertebrates, especially for those that don’t have
very high fertility. Deterministic and stochastic models are playing an
increasing role in population management studies. Although most of the
phytosanitary community is not extensively using tools like this, they
could have usefulness and immediate application. As example, the program
VORTEX3 [13]is probably the most widely used generic PVA model
currently available. It was originally designed for modeling mammal populations,
but is also suitable for modeling birds and some reptiles, invertebrates
and fish populations. The program is individual-based and incorporates
environmental and demographic stochasticity, inbreeding depression, metapopulations,
catastrophes, and some density-dependence functions. The program also allows
for simulation of harvesting from the population and supplementation (i.e.
introducing translocated individuals to the population).
To
show the relation between the population size and the probability of establishment,
we have run few examples in VORTEX, using insect pest data, as is shown
in Figure 9.
Figure 9. Vortex outputs of an insect pest population development,
considering different initial population’s size. A - 500 individual;
B
- 200 individual; C - 100 individual and D - 50 individuals.
As
can be noted from Figure 9 the pest will not establishes if the initial
population is below 200 individuals. The results show also that the probability
of population extinction is 0.29 for an initial population of 500 individual;
0.88 for an initial population of 200 individuals and of 1.0 for initial
populations of 100 and 50 individuals.
This
type of information could support the estimates of the number of individuals
of the pest that could enter the country and, consequently, to supply the
technical justification for the development of safe phytosanitary requirements.
However, the necessary data should be reliable and not always they are
available.
Consignment Size x Frequency of Importations
This
is an issue that is not very often considered as a risk management procedure.
However, the probability of establishment can be greatly changed by frequency
and size of consignments. We had investigated this through a simulation
model representing consignments size equivalent to 100 units per day and
a delivery prevalence of 1% insect pest larvae. The larval mortality is
60%; the pupae mortality is 50% and 50% of the adults mate. The longevity
of the mated adult is around 55 days. Figure 10 presents results of tree
scenarios (a) 1,500 units consignments arriving each 15 days; (b) 3,000
units consignments arriving each 30 days and (c) 6,000 units arriving each
60 days.
Figure
10. Simulation of the development of a population of insects in consignments
arriving in the delivery site at different time intervals. Note that these
results are regarding a fictitious pest.
The
graph in Figure 10 shows that the number of mated individual in the delivery
site can be reduced, through the reduction of the size of each consignment
and reduction of the time interval between the consignments, keeping constant
the yearly amount of the imported product. In spite of this measured being
of difficult practical application, it could represent the difference among
the establishment or not of the pest.
Port of Entry
The
establishment probability is a function of the founder population as well
as environmental conditions. Some techniques used in the study of population
ecology could be used also to support the choice of the risk management
strategies. As an example, Figure 11 [14] below shows a monthly graph of
the humidity versus temperatures in two locations in Brazil. The dashed
square represents the zone of optimum development for Ceratitis capitata
4(16-32oC
and 65-75% RH) as found elsewhere in the literature.
The
graph shows that in Curitiba (temperate south Brazil) the environmental
conditions are sub-optimum all year around, while in Fortaleza (tropical
north Brazil) there are favorable conditions from September to February.
Although it does not allow for any inference on the probability of establishment,
the use of spatial models could be a tool to evaluate the reduction of
the probabilities as function of environmental conditions.
Figure
11. Climogram (Temperature x Relative Humidity) for Fortaleza and
Curitiba, Brazil and zone of optimum development for Ceratitis capitata
(dashed square).
The
country could permit the importation entering in Fortaleza only from March
to August, or only accept Curitiba as the entry port. This measure could
reduce the introduction probability to the acceptable level of risk, as
shown by line a in Figure 6.
There
is commercial software, like CLIMEX5, for modeling species responses
to climate that are been used for quarantine purposes.
4.3
Detection of non-compliant consignments
In
Section 3 it was shown that the number of expected establishments is directly
proportional to the number of entered pests and to the probability of establishment.
The number of entered pests can be decreased by decreasing the infection
rate in the consignments or by detecting consignments with prevalence above
the accepted one.
The
number of samples to be taken from a consignment shall be estimated according
to the hypergeometric distribution (see draft ISPM on Inspection Methodology).
Schilling6 proposed an algebraic equation that approximates
the true hypergeometric distribution. From this approximation we can estimate
the number of samples required to detect a specific infection rate:
9
where
CL
is the confidence level of the sampling and Detection level is the
minimum prevalence to be detected. Using the data from Example 1, in page
7, where the consignment size is 10,000 units and the required detection
level is 0.001 (0.1%) then the sample size would be 2,589 units for a confidence
level of 0.95 and of 3,690 units for 0.99-confidence level.
The
general relation between Detection Level, Confidence Level
and Sample size is presented in Figure 12.
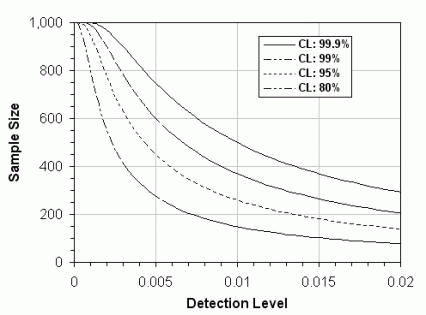
Figure
12. Sample size as function of the detection level, according to the hypergeometric
distribution. The graph shows lines of four confidence levels (CL). The
graph presents the sample size for a consignment of 1,000 units.
Although the
hypergeometric distribution supplies the technical bases for a safe sampling,
it cannot be feasible for very low detection levels, because the number
of units to be sampled would be very high, as can be seen in the Figure
12.
Figure 13. A. Comparison of hypergeometric sampling
with constant size sampling; B. Comparison of hypergeometric sampling
with percent sampling. The graphs were draw considering a detection level
of 0.1% and a confidence level of 95%.
It
is interesting to note that the sampling system used for many countries
is based upon the use of constant size sampling or sampling of a percentage
of the consignment. However, as shown in Figure 13, these systems use either
smaller or larger number of samples than the necessary. In the first case
the safety of the inspection is reduced and in the second case there is
unnecessary resources expenditure.
As
the probability of the inspection to fail is given by 1 –Confidence
Level, it can be calculated from equation 9, accordingly:
10
With the above
equation and the introduction probability, calculated through equation
6, it is possible to verify that a prevalence range exists where the introduction
probability is larger (Fig. 14).
Figure
14. Probabilities of inspection failure, potential introduction and introduction
as a function of prevalence in the consignment (consignment size= 1000,
sample size= 25, probability of establishment= 0.04)
In
this Figure, Potential Introduction means the introduction probability
without consideration of the inspection process and Introduction
is the probability of introduction after the consignment pass through inspection.
The Figure shows that when the prevalence is low the probability of detection
of an infected consignment is also low (if the sampling size is kept constant).
However, when the prevalence increases the probability of detection increases
(decreasing the probability of inspection failure). As the probability
of introduction (before inspection) increase with the prevalence, the probability
of introduction after inspection is bell shaped with a maximum around prevalence
of 2%. It is important to note also that, if the prevalence increases,
the trade would be unviable because the number of refused consignments
would be very high.
5.
Summarizing
The
flowchart for the application of the concepts discussed in this paper is
shown in the Figure 15. The process begins calculating the Acceptable Probability
of Introduction, through the Acceptable Level of Risk (ALR) and of the
potential Economical Impact (EI), with the equation 4. Then, with the equations
5 or 6, and using specific data of the pest and shipment, the Expected
Probability of Introduction is calculated. If the expected probability
is smaller than the acceptable one, then ALR is attained and the trade
could start. Otherwise another measures should be found, either to decrease
the prevalence of the pest or its establishment probability. It could also
be requested smaller consignments. If the expected probability of introduction
is above, but very close to the acceptable probability, the country could
rely in its detection system. In this case it could be expected a larger
number refused consignments.
Figure 15. Conceptual flowchart of the establishment of a phytosanitary
requirement.
Acknowledgments
We
thank to Dr. Ron A Sequeira for the invitation to present this paper in
the International Conference on SPS Risk Assessment Methodology.
We also thank to Dr. Raul Valle for reviewing the manuscript.This work
was accomplished with the supports of the Cocoa Research Center (CEPEC/CEPLAC,
Brazil).
6. References
1.EPPO
- European and Mediterranean Plant Protection Organization. Reporting Service.
1996-2004.
2.
Liquido, N.J., R.L. Griffin, and K.W. Vick (eds.) 1997. Quarantine security
for commodities: current approaches and potential strategies. Proceedings
of Joint Workshops of the Agricultural Research Service and the Animal
and Plant Health Inspection Service, June 5-9 and July 31-August 4, 1995.
USDA, ARS, 1996-04, 56 pp.
3.
Bartlett, P.W., G.R. Chaplin, and R.J. Van Velsend (eds.). 1996. Plant
Quarantine Statistics. A Review. Proceedings of an International Workshop.
Sydney, Australia - December 1995. HRDC, 93 pp.
4.
Bigsby, H. and J. Crequer. 1996. Conceptual model for the management of
pest risk. Statistics in Ecology and Environmental Monitoring, Dunedin,
June 1996, pp. 7-16.
5.
Bigsby, H. and C. Whyte. 2001. Quantifying Phytosanitary Barriers to Trade.
In.: Hooker, N. and E. Murano (Eds.). Interdisciplinary Food Safety
Research, CRC Press, pp. 69-85.
6.
International Plant Protection Convention/FAO. 2001. Pest risk analysis
for quarantine pests. International standards for phytosanitary measures.
Publication n. 11, 27 pp.
7.
Baker, R.T., J.M. Cowley and D.S. Harte. 1993. Pest risk assessment. Lynfield
Plant Protection Centre Publications, New Zealand, No. 1, 12
pp.
8.
Baker, A.C. 1939. The basis for treatment of products where fruit flies
are involved as a condition for entry into the United States. USDA Circ.
551.
9.
Liebhold, A.M., W.L. Macdonald, D. Bergdahl, and V.C. Mastro. 1995. Invasion
by Exotic Forest Pests: A Threat to Forest Ecosystems. Forest Science
Monographs 30.49 pp.
10.
Shaffer, M.L. 1981. Minimum population size for species conservation. Bioscience
31:131-134.
11.
Beissinger, S.R., and D.R. McCullough, editors. 2002. Population viability
analysis. University of Chicago Press, Chicago, Illinois, USA. 577 pp.
12.
Morris, W.F., and D.F. Doak. Quantitative conservation biology: theory
and practice of population viability analysis. Sinauer Associates, Sunderland,
Massachusetts, USA. 480 pp.
13.
Lacy, R.C. 1993: Vortex: a computer simulation model for population viability
analysis. Wildlife Research 20: 45–65.
14.
Araújo, K.R.P. 2000.Modelo matemático para simular a aplicação
da técnica do inseto estéril e etapas de implementação
de um programa de controle da mosca do mediterrâneo. PhD thesis,
CENA/USP, 2001.